Backcasting is Magical
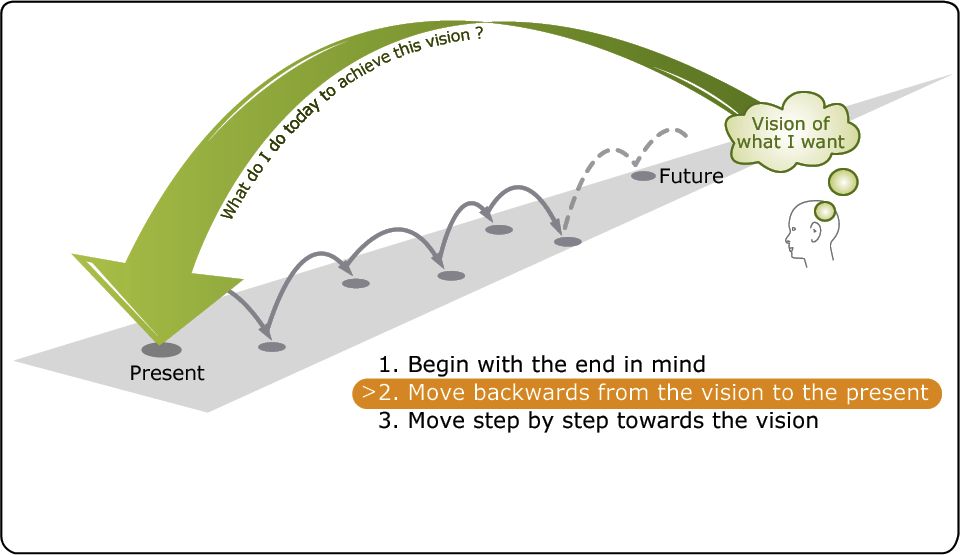
Samim.A.Winiger - 5.5.2025
Traditional logic systems, rooted in the binary true/false dichotomy, break down in the presence of paradox, contradiction, and self-reference. Yet such phenomena are not marginal—they are central to language, consciousness, ethics, and the very foundations of mathematics. Inspired by the Buddhist tetralemma _catuskoti_ and informed by developments in paraconsistent and fixed-point logic, we introduce κ_catuskoti: a meta-logical framework that treats paradoxes as structurally navigable rather than fatal flaws.
κ_catuskoti extends standard logic with four truth values—true, false, both, and neither—allowing it to model contradiction \((P \wedge \neg P)\) and indeterminacy (neither P nor ¬P) natively. A key innovation is the self-reflective operator **ρ**, which enables controlled recursive evaluation of self-referential expressions, including classic paradoxes like the Liar and Russell. We provide a formal syntax, semantics, and computational prototype, and demonstrate the logic’s applicability in reasoning agents, ambiguous ethical scenarios, and meta-linguistic cognition. By parameterizing logical modes with \(\Delta_i\), κ_catuskoti bridges classical, dialetheist, and paraconsistent reasoning. This framework offers a new foundation for logical systems that aim to engage with the complexity of real-world reasoning rather than abstract it away.
_Paradox Computation is simultaneously paradoxical and computational while paradoxically being neither paradoxical nor computational. It computes by not computing and creates paradoxes by resolving them. What is the sound of one hand clapping? Mu!_”
Classical logic falters in the face of paradox, contradiction, and self-reference. These phenomena are not just edge cases; they permeate natural language, cognition, ethics, and computation. Systems that reject or suppress them risk misrepresenting complex, ambiguous realities. κ_catuskoti addresses this gap by building a formal, contradiction-tolerant logic grounded in Buddhist and paraconsistent traditions, capable of modeling self-reference, oscillation, and epistemic uncertainty. This paper presents the κ_catuskoti framework, including its formal syntax and four-valued semantics, the novel self-reflective operator (\(\rho\)) for handling fixed-point paradoxes, and a computational prototype demonstrating its application to challenging reasoning scenarios.
The philosophical background of κ_catuskoti weaves together threads from Buddhist logic, paraconsistent logic, and modern self-referential meta-theories, forming a logic that welcomes paradox rather than eliminating it.
From early Buddhist Madhyamaka philosophy, the _catuskoti_ (Sanskrit: चतुष्कोटि, “four corners") breaks with classical binary logic:
For any proposition P, four possibilities exist:
- P (affirmation)
- ¬P (negation)
- P ∧ ¬P (both)
- ¬P ∧ ¬¬P (neither)
Nāgārjuna, a key philosopher, used this to show the limits of conceptual thought and the emptiness of fixed views. In contrast to Aristotelian logic, which treats contradiction as fatal, _catuskoti_ accepts that some truths are *beyond binary classification*.[^2]
Modern paraconsistent logic—developed by thinkers like Graham Priest—allows contradictions to exist without collapsing the system (avoiding _explosion_, where anything can be proven).
Traditional logic struggles with self-reference:
Most classical solutions involve restrictions or hierarchies (e.g., type theory). But κ_catuskoti, via the ρ operator, embraces self-reference as a native part of reasoning.
ρ allows:
κ_catuskoti arises from a need to:
In essence: κ_catuskoti is not just a formal tool—it’s a philosophical stance: *The world and thought are not always binary. Logic must evolve to match their complexity.*
We begin with a classical logical syntax extended with modal and self-referential operators. The language includes atomic propositions, standard Boolean connectives, and two non-classical constructs:
The grammar of well-formed formulas is defined recursively:
The κ_catuskoti logic operates over a four-valued semantic domain:
Each value corresponds to a distinct logical state:
A valuation function \(v : \Phi \to \mathbb{V}\) assigns each formula a value in this domain.
Let \(\phi, \psi \in \Phi\) and define:
For the definitions of conjunction, disjunction, and implication that follow, the conditions within each cases
block are to be evaluated in order from top to bottom. The first condition that holds true determines the semantic value of the expression.
_(Note: The precise definitions for conjunction, disjunction, and implication in many-valued logics can vary. These represent one possible formalization; specific use cases might warrant alternative definitions, such as those based on lattices like First Degree Entailment.)_
This truth system allows κ_catuskoti to explicitly represent logical uncertainty and paradox. For example, a contradictory claim such as “The court both ruled and did not rule on the case” can be assigned the value \(\mathbb{B}\), whereas a vague or ill-defined claim such as “Justice is red” might be interpreted as \(\mathbb{N}\).
Operators behave intuitively based on the definitions above: Negation flips \(\top\) and \(\bot\), leaving \(\mathbb{B}\) and \(\mathbb{N}\) unchanged. The behavior of conjunction and disjunction prioritizes certain values (e.g., \(\bot\) for conjunction, \(\top\) for disjunction). Implication is defined conservatively.
These design choices maintain non-explosiveness—that is, the logic resists collapse under contradiction, a key feature inherited from paraconsistent principles.
The \(\rho\) operator enables formulas to refer to themselves in a controlled manner. Formally, we define \(\rho(\phi)\) notionally as the fixed point of the operation represented by \(\phi(x)\), where \(x\) conceptually stands for the formula \(\rho(\phi)\) itself. That is, \(\rho(\phi)\) seeks a truth value \(v^{\ast} \in \mathbb{V}\) such that applying the logical operation defined by \(\phi\) to \(v^{\ast} \) yields \(v^{\ast} \) again.
This semantic interpretation is constructed iteratively. We define an evaluation sequence starting with an initial value (e.g., \(\top\) as a convention):
Here, \(v(\phi[v’ / x])\) represents the semantic evaluation of the formula structure \(\phi\) where the placeholder \(x\) (representing the value of \(\rho(\phi)\)) is assigned the value \(v’\) from the previous iteration. The process continues until the sequence of values \(v^{(0)}, v^{(1)}, v^{(2)}, \dots\) stabilizes.
This formalism allows the system to represent and reason about paradoxes internally.
We define the Liar Sentence \(L\) as a formula that asserts its own negation, using the \(\rho\) operator:
Evaluated iteratively, starting with \(v^{(0)}(L) = \top\):
The sequence \(\top, \bot, \top, \bot, \dots\) oscillates indefinitely. According to the rule for stable oscillation:
The \(\rho\) operator formalizes self-reference as a truth-evaluable process. Rather than banning paradoxes like the Liar or Russell’s Set (which would require a set-theoretic formulation), κ_catuskoti models their semantic dynamics directly. This iterative approach aligns with established fixed-point semantics for truth predicates, notably Kripke’s theory of truth (Kripke, 1975), and the dynamic perspective of revision theories (Gupta & Belnap, 1993), which also use stage-based evaluations to handle semantic paradoxes. This enables paradox to be treated as a *computationally tractable phenomenon* rather than a fatal flaw.
Optionally, one can formulate \(\rho\) using category theory by treating \(\mathbb{V}\) as an object in a semantic category, and \(\rho\) as related to fixed-point constructions like those found via coalgebraic methods. This perspective may provide future generalizations.
To handle shifts in perspective or context, crucial for interpreting many koans or evolving knowledge bases, we introduce the context-shifting operator, \(\Delta_i\). This operator is intended to allow the logic to dynamically alter the interpretation or significance of propositions based on a specified context \(i\).
For example, \(\Delta{zen}\) \(( Paradox )\) might evaluate a paradox within a ‘Zen’ context where its contradictory nature is embraced (Value B or N), while \(\Delta{classical}\) \(( Paradox )\) might force a rejection (Value F, depending on underlying assumptions). The \(\Delta_i\) operator represents a mechanism for modulating semantic evaluation based on external or meta-level considerations.
While the intuitive role of \(\Delta_i\) in enabling modal flexibility and context-dependent reasoning is clear, providing its full formal semantics—detailing precisely how it interacts with the valuation function \(v\) and the \(\rho\) operator across different contexts \(i\)—remains a significant avenue for future research. Its inclusion here signals a key direction for extending the core logic’s expressiveness.
Here are three practical examples of the κ_catuskoti logic in action, across different domains where contradiction, indeterminacy, or self-reference must be modeled directly:
Sentence:
“This sentence is false.”
Classical logic:
Breaks due to self-reference:
κ_catuskoti logic:
Encode as a fixed-point, as shown in Section 3.3.2:
\(L := \rho(x). \neg x\)
Evaluate under the four-valued semantics using the iterative \(\rho\) process:
Use case: Formalizing semantics for natural language, analyzing paradoxical statements in text, potential applications in truth valuation for generative models.
Scenario:
An act is judged according to conflicting principles. For example:
“Whistleblowing is both an act of integrity (fulfilling a duty to expose wrongdoing) and an act of betrayal (breaking loyalty oaths).”
Classical logic:
Forces a resolution to either true or false, potentially oversimplifying the dilemma. If represented as \(P \wedge \neg P\), the system risks explosion.
κ_catuskoti logic:
Model the core propositions:
\(P := “The act (whistleblowing) is permissible/good"\)
Assign the contradictory value based on the scenario:
\(v(P) = \mathbb{B}\) (both)
A reasoning system using κ_catuskoti can then use this value:
Use case: Modeling complex ethical dilemmas, AI safety and alignment (handling conflicting values), legal reasoning systems dealing with ambiguous statutes or precedents.
Scenario:
An AI agent needs to represent its own uncertainty about a belief it holds.
“The agent assesses its belief state regarding proposition X as unreliable or indeterminate.”
Classical modal logic: Typically represents belief (\(B(X)\)) or lack of belief (\(\neg B(X)\)), but struggles to natively represent a state of acknowledged indeterminacy about X itself without extensions.
κ_catuskoti logic:
Let \(\phi\) represent the proposition “The agent has conclusive evidence for/against X”.
Allowing propositions to be assigned \(\mathbb{N}\) enables the system to explicitly represent states of doubt, ambiguity, or acknowledged ignorance. This facilitates more nuanced self-reflection and can trigger appropriate behaviors, such as seeking more information rather than acting on an uncertain conclusion. While the \(\rho\) operator _could_ be used for more complex recursive self-assessments (e.g., “I believe that my belief is indeterminate"), the direct use of \(\mathbb{N}\) already provides a powerful tool for representing basic epistemic uncertainty.
Use case: Building more introspective AI agents, modeling cognitive dissonance or uncertainty in cognitive science, developing robust agents for environments with highly uncertain or deceptive information.
While κ_catuskoti is primarily a theoretical framework, its potential computational applications motivate exploring its implementation. A prototype system can demonstrate its feasibility and allow for empirical testing.
A basic prototype has been developed in Python to explore the core mechanics. The evaluation process centers around a function, conceptually evaluate_rho(formula, max_iterations)
, which applies the semantic rules iteratively for self-referential formulas containing \(\rho\). It tracks the sequence of truth values assigned in each iteration up to a predefined limit (max_iterations
) to determine convergence (to T, F, B) or divergence (assigned N). Standard test cases, such as the Liar Paradox formulated as L: \rho(\neg L)
, were used to validate the mechanism, confirming its ability to assign the expected paradoxical value (B in this case, assuming convergence within the iteration limit).
The engine includes implementations for:
The prototype allows simulating how an AI agent equipped with κ_catuskoti logic might handle contradictory or indeterminate information. Consider an agent evaluating safety conditions:
This simulation highlights how κ_catuskoti enables nuanced decision-making, allowing agents to recognize and react appropriately to contradiction and indeterminacy rather than defaulting to arbitrary choices or system failure.
The κ_catuskoti framework, with its capacity to handle contradiction, indeterminacy, and self-reference, opens significant applications across diverse fields.
In essence, κ_catuskoti provides a foundation for systems that engage more directly with the paradoxical and ambiguous nature of real-world information and reasoning processes, moving beyond the limitations of purely binary logic.
To situate κ_catuskoti within the landscape of existing logical frameworks, it is useful to compare its capabilities against related systems. Notably, the four-valued semantics employed here share the same truth values \({\top, \bot, \mathbb{B}, \mathbb{N}}\) as Nuel Belnap’s influential logic of First Degree Entailment (FDE), often used in computer science to handle inconsistent and incomplete information (Belnap, 1977).
However, while sharing this foundational structure, κ_catuskoti introduces crucial distinctions:
The following table summarizes key feature differences across several logical paradigms:
Logic System | Contradiction | Indeterminacy | Self-Reference | Dynamic Semantics |
---|---|---|---|---|
Classical Logic | ✘ | ✘ | ✘ | ✘ |
Fuzzy Logic | ✘ | ✓ | ✘ | ✘ |
Paraconsistent Logic | ✓ | ✘ | partial [^1] | ✘ |
Modal μ-calculus | ✘ | ✘ | ✓ | ✓ |
κ_catuskoti | ✓ | ✓ | ✓ | ✓ |
While the κ_catuskoti framework presented here offers a novel approach to handling paradox and inconsistency, it represents foundational work with several avenues for further development. We identify the following key limitations and directions for future research:
Addressing these areas would further mature κ_catuskoti as both a theoretical framework and a practical tool for advanced reasoning systems.
κ_catuskoti logic offers a radical yet rigorous reconceptualization of logical foundations: one that embraces contradiction, supports indeterminacy, and renders paradox productive. By synthesizing Buddhist tetralemmic thought with modern fixed-point meta-logic and four-valued semantics, this system reframes long-standing philosophical and computational limitations. The introduction of the \(\rho\) operator allows for dynamic, recursive truth evaluation, making κ_catuskoti uniquely suited for domains where self-reference and epistemic instability are the norm, not the exception.
Our computational prototype demonstrates how an AI agent using κ_catuskoti can reason coherently in contradictory environments—a capacity increasingly relevant for systems interacting with complex, conflicting human data. This logic not only resists the collapse of classical systems under paradox but transforms paradox into an epistemic resource. Looking ahead, κ_catuskoti opens promising avenues for machine consciousness, legal and moral pluralism, and robust epistemology under uncertainty. In rejecting the tyranny of the excluded middle, it brings logic one step closer to the way real minds—and real worlds—work.
For further context and related topics, readers may find the following resources and areas of exploration helpful:
Yablos Paradox is the idea that there is no way to coherently assign a truth value to any of the sentences in a countably infinite sequence of sentences, when these sentences all state that “all of the subsequent sentences are false”.
"The point of these observations is not the reduction of the familiar to the unfamiliar[...] but the extension of the familiar to cover many more cases." - Saunders MacLane, Categories for the Working Mathematician, Page 226
Most truths cannot be expressed in language
The Period-doubling cascades en route to chaos remains one of the most puzzling features of nature
Was Floquet Engineering to identify nonlinear resonances in Floquet‑Driven Reservoirs all night long. Exhausted but unbothered. moisturized. happy. in my lane. focused. flourishing. periodically driven.
The authors of the study suggest that the brain uses the superposition & interference patterns of waves to represent and process information in a highly distributed way, exploiting the unique properties of coupled oscillator networks such as resonance and synchronization.
Code: Harmonic oscillator recurrent network (HORN) Pytorch Implementation
Recast neural processing as continuous‐time dynamics rather than pure feed-forward stateless layers.
Embedding physical, continuous dynamics—oscillations, waves, vortices, and damping—directly into the network’s layers unlocks powerful, implicit mechanisms for attention, multi-scale integration, and long-range memory, without the combinatorial bloat of explicit attention heads or deeper stacks.
Damped Harmonic Oscillator (DHO) nodes
In model.py
, each hidden unit integrates its “position” x and “velocity” y via:
Key Insight: forcing every node into an oscillatory regime makes phase, frequency and amplitude available for coding from the very first forward pass—no need to “discover” oscillations through recurrent loops alone.
Why it matters:
Traveling waves & standing waves
High‐Dimensional Mapping:
h2o
layer.Code touch-point: The record=True
flag in forward()
gives you rec_x_t
and rec_y_t
. Visualizing these (as in dynamics.py
or train.py
) shows exactly how different input classes sculpt different wave‐interference signatures.
The intrinsic gain curve of each DHO node is defined as:
In the PNAS work, a grid‐search found optimal \omega \approx 2\pi/28 to match the dominant frequency of straight strokes in sMNIST.
Computational payoff: Nodes automatically amplify (resonate) in-band inputs—so you’re baking in priors about the signal’s spectral content at the architectural level, not just via learned weights.
How to explore in your code:
dynamics.py
, drive a single DHO with a pure sine wave at different frequencies.Biological motif: neurons/pyramidal microcircuits have tunings and delays that vary across the network.
HORN’s version: drawing each unit’s \omega_i and \gamma_i (and later, delays) from a distribution.
Why it helps:
Simple test in code:
dynamics.py
you already sample omega
and gamma
. Try:
rec_x_t
snapshots and compare classification accuracy.Surprising finding: gradient‐based BPTT weight updates on h2h
end up correlating strongly with pre-post activity correlations—i.e. they “look Hebbian.”
Takeaway: Even though you’re minimizing a cross‐entropy loss at the readout, the recurrent weight updates reorganize the network to amplify stimulus‐specific synchrony patterns.
Next step in code:
Biological signature: cortex at rest floats through a “rich club” of states; stimuli transiently collapse it into a low‐variance, stimulus‐specific subspace.
HORN mimic:
y_t
(Poisson noise) when record=False
Weekend immersed in Deep Oscillator Neural Networks has been a resonant journey.
Phase-encoded information is elusive: no single wave holds meaning. It’s the alignment, the relationship, that reveals it. It dominates in systems across nature where precision, interference, or efficiency matter.
Nivida - Microsoft Revenues Round Tripping scheme here in all its beauty
In case you wanted any proof of $MSFT round tripping revenues at a massive scale in order to inflate its financial results here you have it right out its own last 10-Q
Kaznacheyev effect
Introduction: Ever thought cells could communicate like a cell phone but with light? Enter the fascinating and controversial realm of the Kaznacheyev effect!
The Discovery: In the 1970s, a Russian scientist named Vlail Kaznacheyev, along with his colleagues, stumbled upon a phenomenon that would spark decades of debate. They observed that cells don’t go quietly into the night; instead, as they perish, they emit ultraviolet (UV) photons, like desperate SOS signals.
The Experiment: Kaznacheyev’s team placed two sets of cell cultures close to each other, separated only by a quartz barrier that let UV light pass through. When one set of cells was inflicted with doom (think viruses, toxins, or harmful radiation), these dying cells reportedly sent out UV light signals. The astonishing part? The neighboring cells, previously healthy, started showing signs of the same fate as if the light carried a morbid message.
The Twist: A glass barrier instead of quartz? The second set of cells remained unaffected, living their best cellular lives. It seemed that the UV light, with its mysterious cargo, couldn’t pass through glass, halting the transmission of the deadly message.
The Controversy: Dubbed the “cytopathogenic effect,” this phenomenon suggested something revolutionary—that diseases could potentially be transmitted electromagnetically. But here’s the catch: science thrives on replication, and the Kaznacheyev effect has been notoriously shy in other labs.
The Implications: If the effect is real, it could hint at an intricate bio-communication system and electromagnetic influences on health, a topic that’s gaining traction in today’s tech-filled world.
The Fun Fact: While the scientific jury is still out on the Kaznacheyev effect, it opens up a world of sci-fi-esque possibilities. Could our cells be gossiping about their demise? Are they warning their neighbors through a UV light group chat? The ideas are as intriguing as they are controversial.
Whether a quirk of experimental conditions or a genuine biological phenomenon, the Kaznacheyev effect reminds us that there are still mysteries within our own cells waiting to be understood. It’s a cellular conundrum that keeps the conversation glowing—quite literally!
Conclusion: Vlail Petrovich Kaznacheyev was a Russian biologist and a known figure in the field of biophysics. He is most recognized for his work related to the controversial phenomenon known as the Kaznacheyev effect, which he claimed to have discovered in the 1970s alongside his colleagues. According to his research, cells that were dying emitted ultraviolet (UV) photons, and these photons could transmit the “information” of cellular death to neighboring cells, causing similar effects in those cells if they were in quartz containers that allowed the passage of UV light. If a barrier that blocked UV light, such as glass, was used, the effect was not observed.
Regarding the proof to back up the Kaznacheyev effect, it’s important to note that this phenomenon has been met with skepticism within the scientific community. One of the main criticisms is the lack of independent replication of his results, which is a cornerstone of scientific validation. The experiments conducted by Kaznacheyev and his team were indeed numerous, but the methodology and the results were not widely accepted or reproduced by other researchers.
The idea that cells could communicate distress through electromagnetic signals, especially in the form of light, is certainly fascinating and has implications for our understanding of cellular processes and disease transmission. However, the evidence supporting the Kaznacheyev effect is not robust within the mainstream scientific literature.
There are fields of study such as bioelectromagnetics and biophotonics that explore the role of electromagnetic fields and light in biological processes, and some research within these disciplines has indicated that cells can emit light (biophotons) and that these emissions can vary with the state of the cell’s health. However, whether these emissions can induce pathological changes in other cells as described by the Kaznacheyev effect remains unconfirmed.
"Time saved by AI offset by new work created, study suggests"
By now everyone could be living on a 2-day workweek in fully automated luxury - but instead we’re slaving away at bullshit jobs harder than ever. The game’s rigged.